Appraisal of Credit Applicant Using Logistic and Linear Discriminant Models With Principal Component Analysis
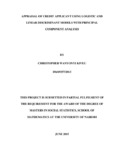
View/ Open
Date
2015Author
Kiveu, Christopher W
Type
ThesisLanguage
enMetadata
Show full item recordAbstract
In this project, we examine the criteria for classify credit applicant status using Binary Logistic Regression and Linear Discriminant models with Principal Components as input variables for predicting applicant status in terms of Creditworthy or Non- creditworthy. Information collected for previous credit applicants is used to develop the models for predicting the new applicant’s creditworthiness. The results obtained showed that the use of Credit factors obtained from Principal Components as input variables for Linear Discriminant (LDA) and Logistics Regression (LR) models prediction eliminated data co-linearity and reduced complexity in dimensionality by grouping variables together with little loss of information. Based on Eigen values with values above 1, seven factors were retained. The factors accounted for 76.09 percent of the total variation. One thousand credit applicants were considered; 715 as creditworthy and 285 as un-creditworthy. The result obtained from the analysis showed that Logistic Regression gave classification accuracy 87% slightly better than discriminant analysis 85.60%. However, discriminant analysis achieved less cost of misclassification 48 than Logistic regression 72 for non-creditworthy applicants classified from the 1000 applicants.
Rights
Attribution-NonCommercial-NoDerivs 3.0 United StatesUsage Rights
http://creativecommons.org/licenses/by-nc-nd/3.0/us/Collections
The following license files are associated with this item: